The Transformative Power of Netflix Recommendation Algorithm

Let’s peel back the curtain on a hidden gem that has revolutionized our viewing experience - the Netflix recommendation algorithm. This ingenious creation has one clear purpose: to understand, anticipate, and cater to the diverse palates of Netflix users. The platform’s user base is a vast tapestry interwoven with individuals of all age groups, hailing from different corners of the globe. Each viewer’s unique preferences, be it gripping Korean dramas or light-hearted stand-up comedies, are all taken into consideration. Drawing on the fundamentals of machine learning and data analysis, and amplified by artificial intelligence, the Netflix recommendation algorithm masters the art of making strikingly personalized content suggestions. This paves the way for undervalued content to surface and previously unknown content to be discovered. As we dive into the labyrinth of this algorithm, remember one thing: it’s all about personalization. In essence, it's a tale of how data is transformed into a cinematic journey crafted just for you.
Understanding Netflix and its User Base
Netflix, a well known global streaming platform, hosts a diverse user base of approximately 214 million paid subscribers spanning across 190 countries. The age range of these viewers is incredibly varied, from younger audiences appreciating animated content to elders indulging in dramatic or documentary-style content. A survey conducted by Statista in 2020 demonstrated this well-roundedness, revealing that 15% of users were between the ages of 18 and 29, 29% were in their 30s and 40s, and the remaining audience was spread across older age groups.
Additionally, geographic location largely influences the content preferences for Netflix users. For instance, while users in the United States showed a predilection for sitcoms like ‘Friends’ and ‘The Office’, global viewers displayed a strong preference for diverse content including Korean dramas, British mysteries, and Scandinavian crime thrillers, according to a study by HighSpeedInternet. Language, too, plays a pivotal role. With features allowing content streaming in over 20 languages, Netflix has indeed transformed itself into a global content library. From the romance of Spanish drama ‘Money Heist’ to the thriller of German series ‘Dark’, Netflix’s spectrum of content satisfies an array of linguistic preferences among its users. Therefore, the Netflix recommendation algorithm has the imperative task of catering to this extensive and sundry audience base, considering numerous factors such as age, location, genre, and language.
The Science Behind Netflix Recommendation Algorithm

Netflix's recommendation algorithm fundamentally operates using machine learning and data analytics principles. Machine learning employs a series of algorithms to analyze user patterns, learn from them, and predict future behavior. For instance, if a user frequently watches science documentaries, the algorithm learns this preference and suggests similar content. Data analytics, on the other hand, involves examining raw data to draw conclusions. For Netflix, this could mean analyzing the viewing patterns of millions of viewers to identify commonalities and divergences, enabling more accurate content curation.
Furthermore, artificial intelligence (AI) plays a significant role in enhancing the algorithm's performance. AI technologies are used to refine the machine learning algorithms, enabling them to learn more complex viewing patterns and offer more sophisticated recommendations. This approach ensures the system becomes better over time, constantly learning and perfecting its ability to predict viewers' preferences. For instance, the system may infer from user behavior that people who enjoy action films also usually enjoy thrillers.
Hence, Netflix leverages a combination of machine learning, data analytics, and AI to curate a personalized viewing experience for its audience. Its recommendation algorithm is an exemplary representation of how these technologies can transform traditional viewing habits, shifting from a one-size-fits-all approach to personalized content curation. Rather than viewers looking for content, the content finds the viewers.
How the Netflix Recommendation Algorithm Works
With Netflix's extensive reservoir of content, finding a movie or show that aligns with an individual's preference can be a challenge. This is where Netflix's recommendation algorithm comes into play—tailoring content selection to personal taste and behavior. It begins with understanding the user's previous activity—everything, from the shows they've watched to how long they watched them. This interaction history forms the foundation for subsequent recommendations.
Ensuring users discover new and potentially overlooked content is a huge part of its function. As users interact with the Netflix platform, the algorithm adapts, generating suggestions that deviate from their usual watch patterns. For instance, if someone has been watching crime thrillers consistently, the system may suggest a critically acclaimed drama based on positive response from a similar viewer demographic. This not only exposes the user to a broader range of content but also gives lesser-known shows a chance to be discovered.
Taking it a step further, the algorithm promotes new content based on behavioral data from vast numbers of subscribers. If a new comedy series generates significant traffic, the algorithm may push it to users who favor comedies even if they haven't viewed much of the show's type beforehand. This is a prime example of how Netflix promotes fresh and diverse content, keeping subscribers' interests piqued.
Besides specific behavioral data of an individual, the algorithm also considers aggregated data of users with a similar watch pattern. Suppose a large portion of users who enjoyed a certain documentary also highly rated a particular sitcom. The algorithm uses that data point to nudge the sitcom to other viewers of the documentary, cross-pollinating tastes and recommendations.
In a nutshell, the Netflix recommendation algorithm is a user-centric, data-driven machinery that constantly learns from user activity to tailor and enhance the viewing experience. It takes the guesswork out of searching for what to watch next, somewhere between catering to users' tried-and-true tastes and nudging them towards new content horizons. It is a major factor in Netflix's success, serving individual entertainment on a mass scale.
Algorithmic Personalization and its Impact on User Experience

The effectiveness of Netflix's recommendation algorithm cannot be overstated, particularly when it comes to enhancing the user's viewing experience. Personalized recommendations, driven by complex algorithmic intelligence, allow users to seamlessly navigate through a vast array of content. It curates a tailored library matching individual's inclined genres, styles, or themes, saving indecisive moments and streamlining the selection process. For instance, if a user frequently watches crime dramas, they will find more similar shows populating their recommendation list, providing an uninterrupted entertainment experience.
An overlooked yet crucial advantage of the algorithm is its potential in uncovering new content. With hundreds of thousands of shows and movies, many quality titles can get buried or unnoticed. By closely studying and analyzing the viewing habits, genres liked, watch times, and other minute intricacies, the algorithm can promote undiscovered gems well suited to a user's taste that might otherwise remain overlooked. Bridgerton, for instance, was suggested to a significant number of viewers who previously engaged with historical dramas or romance genre content, turning it into a global phenomenon.
Amongst a diverse audience with vast preferences, the algorithm plays a significant role in content discovery too. Users can effortlessly encounter new content aligned with their tastes, genres, and inclinations, peek into different cultures via international content, or even challenge their comfort zone with diverse recommendations. It's like having a personal companion who knows your tastes and constantly broadens your horizon by introducing exciting shows and films. This is why despite an initial public skepticism towards "The Witcher," the algorithm's strategic suggestions through connections with other action and fantasy titles led to it becoming one of Netflix's most successful originals.
Data Analysis: The Foundation of Netflix Recommendation Algorithm
The importance of data analysis in the operation of the Netflix recommendation algorithm cannot be understated. Every streaming session by users generates a wealth of activity data, which Netflix captures and ana lyzes in real-time. This raw data provides a rich tapestry of user preferences, habits, and other valuable insights from which Netflix can craft highly personalized recommendations. Every movie clicked, every series binged, and even the time spent hovering over specific content, when aggregated and dissected, become invaluable in delivering tailored user experiences.
Netflix's data analysis phase is akin to mining gold from an untapped mountain. It takes this mountain of raw data and begins the process of extracting the nuggets of wisdom that form the basis of its recommendations. This process involves a sophisticated transformation of raw data into actionable insights. For instance, data concerning a user's preference for romantic films, drawn from their viewing history, may lead to the suggestion of a romantic series of similar thematic elements. Every piece of analyzed data is used to enhance Netflix's ability to serve content that synchronizes with individual viewer preferences, ensuring a more engaging and satisfactory user experience. Without this rigorous data analysis, the elaborate personalization the algorithm achieves would be practically unattainable.
Diving Deeper into Machine Learning Aspects
The Netflix algorithm heavily relies on unsupervised machine learning methods which, unlike their supervised counterparts, do not require training data with correct answers. These algorithms identify patterns in data by themselves, optimizing the model without human intervention. For example, clustering algorithms, a type of unsupervised learning, are utilized by the Netflix recommendation system to group similar users or movies together based on the viewing patterns.
Also integral to the machine learning component of Netflix's recommendation system is collaborative filtering. This technique enables highly personalized recommendations by analyzing past behavior of multiple users in addition to the viewer's own behavior. Rather than purely focusing on the content specifics, it allows the system to suggest movies that “similar” users have watched and liked. Hence, if given viewers have enjoyed the same films in the past, it is likely that they are going to agree on other films too.
Collaborative filtering works in two primary ways, user-based and item-based. User-based filtering compares the user in question to all other users, searching for users with similar viewing habits. It then suggests films that these similarly-minded users have appreciated. Item-based collaborative filtering, on the other hand, compares items that the user has already rated and recommends items that are similar to those.
Microsoft Research suggests that collaborative filtering is best for providing personalized recommendations. In line with this, Netflix utilizes both user-based and item-based collaborative filtering to create a dynamic system of recommendation. These methods successfully cater to the fluidity of viewer preferences, providing a solution that evolves with user behavior.
To sum it up, through unsupervised learning methods and collaborative filtering, Netflix delivers intuitive and highly tailored viewing recommendations. This contributes significantly to the service's overall appeal, enhancing user experience by consistently providing suggestions that align with individual tastes.
A/B Testing: Perfecting the Recommendation Experience
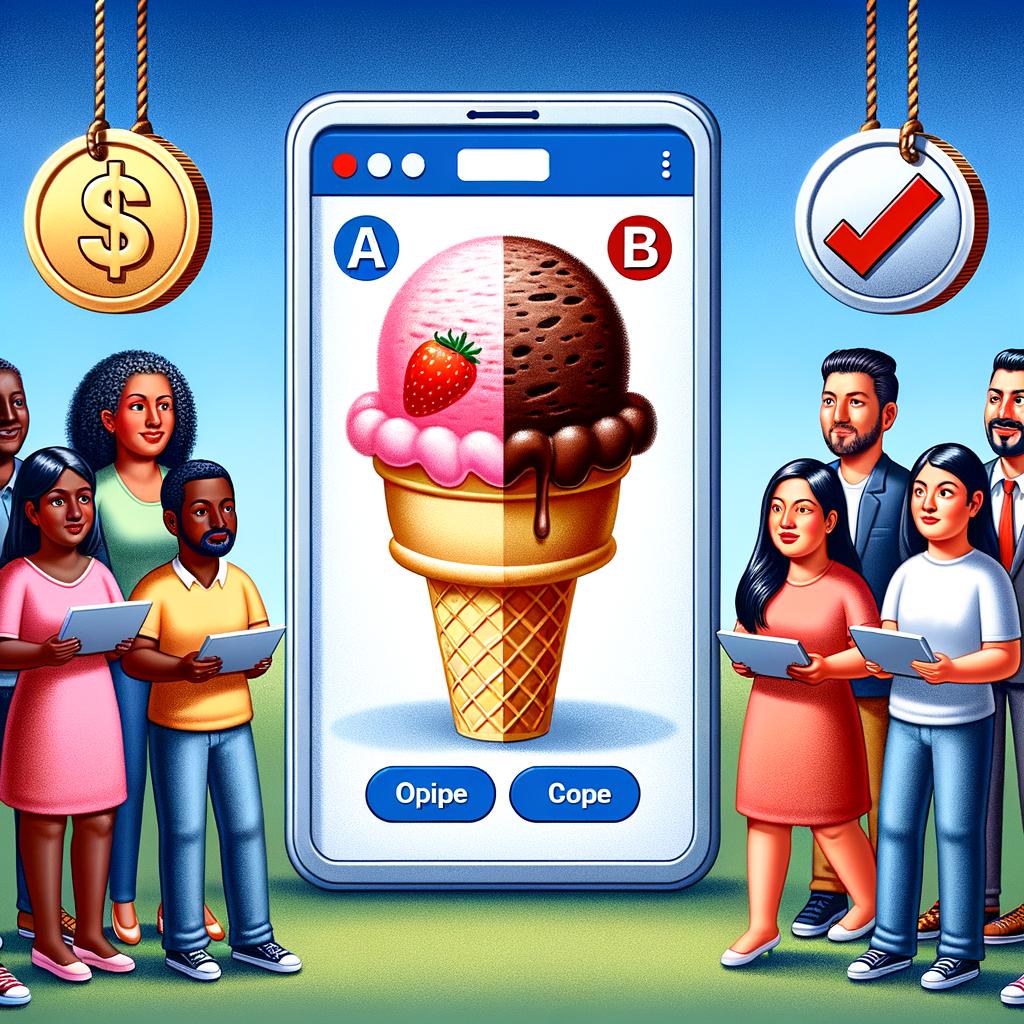
A/B testing fundamentally propels the Netflix recommendation algorithm to its zenith, ensuring the user experience is ever-improving and memorable. One of the central ways A/B testing has been instrumental regards the aspect of refining algorithm performance. Through continuous trials, contrasting one strategy or variation against another, it has been an integral part of Netflix's iterative improvement process.
Examples abound as to how A/B testing has helped Netflix fine-tune its algorithm to better cater to every user's unique preferences. Take, for instance, the strategic adjustment of recommendation mechanisms to use language, actors, or directors as recommendation points. This was implemented after results from A/B testing highlighted a tendency for users to gravitate toward content associated with familiar names and languages.
Furthermore, there's evidence of A/B testing's enormous impact on the visual aspect of content recommendations. Netflix experimented with different variations of thumbnail displays for its content which, in turn, directed subsequent decisions to feature dynamic and character-specific thumbnails. Users were found to respond positively to relatable images, making interaction with the platform more engaging and enticing.
In other instances, changes in recommendation categories have been directly influenced by A/B testing insights. Subtle adjustments such as reordering content according to user behavior patterns, or redesigning UI elements for improved user navigation, are implemented after rigorous testing. This allows the platform to enhance and simplify the content discovery process for its users, therefore providing an increasingly personalized and enjoyable viewing experience.
Finally, A/B testing amplified the algorithm’s ability to recommend newer and overlooked content. This approach has resulted in the discovery of unexpected user preference patterns, unveiling content that would otherwise go unnoticed. By leveraging A/B testing, Netflix has carved out a niche in uncovering hidden content, adding depth to its user experience.
In essence, A/B testing is unequivocally integral to improving Netflix's recommendation algorithm, shaping the way we consume media in a uniquely customized and organic manner. It not only aids in refining the algorithm's functionality but also betters the overall content viewing and discovery experience for the user.
Assessing the Accuracy of Netflix's Algorithm
Netflix's recommendation algorithm's accuracy is rigorously tested using multiple tools and metrics, enabling a comprehensive and precise assessment. Metrics, such as precision at k (P@k) and normalized discounted cumulative gain (NDCG), play an integral role in validating the accuracy of predicted recommendations. These metrics allow Netflix to determine whether the algorithm is indeed suggesting content that users will appreciate and enjoy.
Crucially, the Netflix team carried out a detailed accuracy assessment and produced informative results. Their findings demonstrated that the recommendation algorithm has a considerable degree of proficiency in aligning with user preferences. This is evident from the fact that a considerable percentage of people watch the suggestions made by the algorithm daily.
Moreover, some studies suggest that the percentage of users watching recommended content increased by up to 75% in recent years. Supporting this, a research paper published by Carlos A. Gomez-Uribe and Neil Hunt implied that the recommendation system's successful prediction rate substantially boosted subscriber numbers.
Indeed, captivating anecdotes like these reaffirm the effectiveness of Netflix's recommendation algorithm while highlighting its colossal influence on user engagement. Netflix's relentless pursuit for precision shows its undying commitment to enhancing the user experience.
The motive behind Netflix's persistent efforts lies in their belief that better recommendations provide superior user experience and thereby, assure user retention. Thus, the accuracy assessment results reinforce the algorithm's success in accurately identifying and promoting films and TV shows based on individual user preferences.
How Netflix Recommendation Algorithm is Shaping Viewing Habits
Netflix's recommendation algorithm has been instrumental in molding the viewing practices of its users, encouraging a phenomenon known as "binge-watching." This term describes the user's habit of continuously watching an entire season or series of a show in one or a few sittings, thanks to the algorithm suggesting the "next episode" of a series based on their current viewing activity. This has led to drastic shifts in audience consumption trends and even impacted cultural experiences of watching TV, making binge-watching a common practice among Netflix users.
The Netflix algorithm also significantly shapes user preferences over time. As it keeps suggesting and promoting content similar to what the user has previously watched or liked, viewers tend to explore and often grow fond of genres and themes they might initially be unfamiliar with or even averse to. For example, a user who typically watches thrillers but tries a comedy show on a whim might start receiving more recommendations for comedies, broadening their TV viewing habits over time.
Moreover, the recommendations serve more than just meeting individual preferences. They also highlight overlooked or lesser-known content that matches a user's watch history, driving more diverse viewership. Netflix, through its algorithm, is essentially influencing not just what shows or movies a viewer watches, but also how and when they watch them, and what they might watch next, evolving the overall experience and trend of on-demand viewing. The daily viewing habits of millions are thereby subtly reshaped, influencing global TV consumption habits and setting a precedent for other streaming platforms.
Netflix's Steps to Improve Algorithmic Accuracy

Netflix's recommendation algorithm has been under constant development since its inception. The company understands the significant role that this tool plays in maintaining and enhancing the user experience. Thus, they have consistently made improvements aimed at increasing the algorithm's accuracy over the years. For instance, through hosting public competitions, Netflix has tapped into external expertise to gain fresh insights.
Netflix has set its sights on certain key areas for future improvements as well. The firm intends to focus on bolstering the algorithm's ability to accurately predict a user's taste. This underscores the goal of delivering a more personalized viewing experience. Enhanced personalization could help unearth underappreciated content that aligns with individual user's tastes but might have previously been overlooked.
Advancements in technology serve as a beacon for potential enhancements. Particularly, the integration of emerging AI techniques like deep learning into the algorithm could be a game-changer for Netflix. The heightened predictive capabilities of AI promise to hone the accuracy of content recommendations.
Admittedly, Netflix will encounter fresh challenges as it forges ahead with the refinement of its algorithm. Notably, the 'cold start problem', where the algorithm struggles with making accurate recommendations due to insufficient user data, remains a considerable challenge. Further, the company should avoid the issue of modeling the recommendation algorithm too closely to existing data patterns, commonly known as 'overfitting'.
Yet, despite potential hurdles, Netflix remains optimistic about the prospects. Their diligent approach to overcoming challenges further solidifies their commitment to continually enhancing the algorithm's accuracy. As Netflix continues to evolve, the advancements in its recommendation algorithm appear to be a vital part of its growth strategy.
Ultimately, the trajectory of Netflix's recommendation algorithm reflects a commitment to user satisfaction. By persistently enhancing and refining its algorithm, Netflix continues to optimize the viewing experience for users globally. This meticulous approach to algorithmic improvement is testament to Netflix's dedication towards superior streaming experience for its subscribers.
How User Feedback Enhances the Algorithm's Effectiveness
User reviews and ratings play a critical role in enhancing the effectiveness of Netflix's recommendation algorithm. These elements work as a feedback system, providing input that allows the algorithm to refine its recommendation process. For instance, if a user rates a series highly, the algorithm considers this data and enhances their viewing experience by suggesting similar genre or theme-based content, thereby improving the personalization of its recommendations.
The feedback loop mechanism employed by Netflix stands as a powerful tool in increasing the algorithm's accuracy. This dynamic system ensures that the algorithm is continually learning and evolving to serve user preferences more accurately. It's akin to a real-world conversation, where one person's response significantly influences the future course of the conversation, retaining an element of dynamism. Through consistent user engagement and feedback, Netflix's recommendation algorithm is thus able to maintain its relevance and effectiveness, and prove its worth by creating a more enriched and custom-tailored viewing experience for each user.
Comparing Netflix's Algorithm with Other Streaming Platforms
Distinguishing Netflix's recommendation algorithm from those of other streaming platforms like Amazon Prime and Hulu offers an enlightening perspective. Netflix's algorithm stands apart manly due to its unique features which have been gradually added and refined over time. The algorithm's focus on specific viewer preferences to provide targeted recommendations is unparalleled in the industry. This allows Netflix to guide viewers to content they're more likely to enjoy, enhancing customer satisfaction and prolonging viewer engagement.
In contrast, platforms such as Hulu and Amazon Prime, utilise a more generalized approach, leaning more towards popular trends and ratings. For instance, while Netflix meticulously studies your viewing behavior and serves a selection that aligns with your preference, Amazon Prime would heavily emphasize content that has amassed good reviews or generated a lot of buzz.
Surpassing competition, Netflix integrates feature such as usage of unsupervised learning methods and a unique collaborative filtering technique. This results in a more personalized recommendation, leading to an user-oriented experience. It's the fine tuning of such powerful attributes that puts Netflix a step ahead, illustrating how essential the recommendation algorithm is to the platform's success.
Challenges Faced by Netflix in Improving the Algorithm
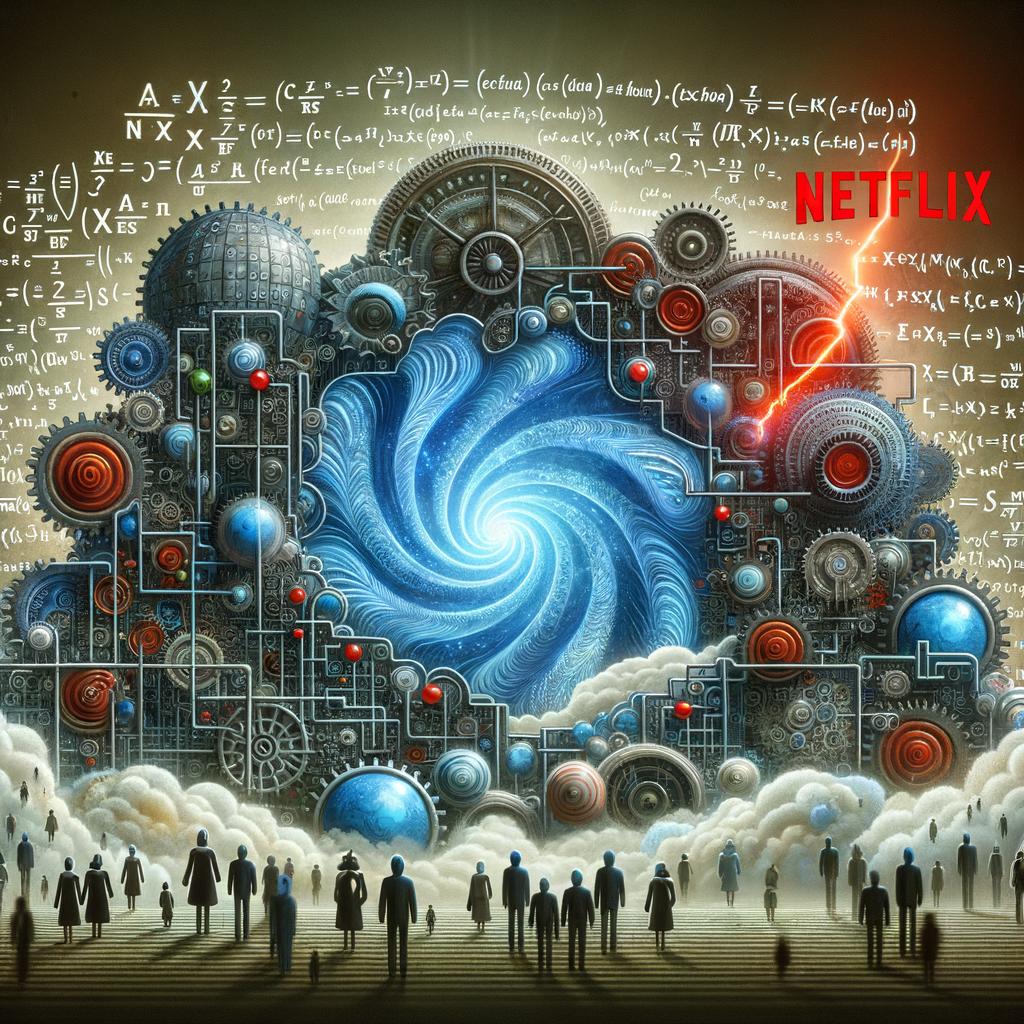
Improving Netflix's recommendation algorithm entails overcoming several key challenges. Firstly, the "cold start" problem arises with new users who have yet to stream content. With no history to analyze, the algorithm struggles to provide pertinent suggestions. To address this, Netflix prompts new viewers to rate sampled programs, aiding the algorithm in generating starter recommendations.
Overfitting is yet another issue. This occurs when the algorithm becomes too specific, fitting limited data samples and disregarding wider trends. As a result, the recommendations may become niche, underrepresenting a user's varied interests. Netflix combats this by continually refining their machine learning methods.
It's crucial to note that advancements in the algorithm impact not only Netflix but also the wider streaming industry. While striving for improvement, Netflix inadvertently sets the bar higher for other streaming services. The lifted standards translate into more accurate viewer recommendations industry-wide.
Balancing novelty and familiarity is another formidable challenge. Netflix must avoid defaulting to safe recommendations and ensure overlooked content gets the spotlight. The algorithm's goal extends beyond merely suggesting what viewers will like; it should also introduce content that pushes viewers out of their comfort zones.
Finally, managing user satisfaction while implementing changes is a tightrope task. Any changes in the algorithm can potentially disrupt the user experience. Hence, Netflix adopts a careful, incremental approach while refining the algorithm's performance.
The Broader Implications of Netflix's Recommendation Technology
Netflix's recommendation algorithm plays a pivotal role in sculpting the landscape of online media consumption. This novel technology has the potential to be leveraged beyond the sphere of content streaming and into other domains, offering a myriad of possibilities. For instance, it could be applied to e-commerce or social media platforms to suggest products or posts based on a user's activity, essentially personalizing their experience. Furthermore, with the ongoing advancement in data analytics and artificial intelligence, Netflix's groundbreaking algorithm also serves as a blueprint for future innovations, demonstrating the transformative power and the vast potential of well-conceived, data-driven technologies.
The Pros and Cons of Algorithm-Driven Recommendations
Algorithm-driven recommendations have many benefits, aiding in user retention and content discovery. By providing tailored suggestions, it maximizes user engagement. This personal touch keeps people coming back, eager for new, carefully curated content. For example, someone who enjoys true crime documentaries will find more of the same genre in their feed, encouraging more viewing.
Still, while binge-watchers might enjoy the continuous stream of preferred content, it may prove limiting, restricting exposure to diverse offerings. Personalized recommendations could potentially create a "bubble" effect, confining viewers to a specific content type.
Furthermore, the issue of privacy comes to the fore. Collecting data to personalize recommendations can lead to concerns about user privacy. Although Netflix states that data collected solely impacts viewing experience, privacy remains a concern for many, mindful of the information shared online.
Despite these challenges, there's an undeniable positive - enhancement of content discovery. Previously overlooked series or films now stand a chance of being discovered thanks to this algorithm. A previously obscure indie film may suddenly gain tremendous viewership, only because the algorithm suggested it to the right audience.
However, there are limitations in the algorithm's accuracy. While it generally works well, it may miss the mark occasionally, suggesting a rom-com to a sci-fi enthusiast. These drawbacks highlight the algorithm's reliance on past behavior, potentially skewing its predictions.
In summary, while algorithm-driven recommendations undoubtedly have their upsides in user retention and content discovery, they also present challenges, such as potential privacy concerns and accuracy limitations.
Netflix's Future: What's in Store for the Recommendation Algorithm?

Looking ahead, Netflix has outlined ambitious aims for the betterment of its recommendation algorithm. They are keen on further incorporating AI advancements, such as deep learning, to refine the algorithm and enhance user experience. Deep learning, a subset of machine learning, possesses the capacity to mimick the human brain in processing data for decision making, which could be pivotal in shaping user-specific recommendations. For example, predicting a user's preference for a movie genre based on their history of movie watches, search history, and demographic data. With such potential enhancements, the viewer's experience on Netflix could become even more personalized and engaging.
Conclusion: The Transformational Influence of Netflix's Algorithm
This transformative algorithm has certainly reshaped online media consumption, giving viewers a highly personalized experience while inciting discoveries of new content. As Netflix continues to refine and enhance its algorithm, the outcome will likely lead to even more significant influences within the realm of digital entertainment. The potent blend of technological progression and viewer satisfaction signify a dynamic and groundbreaking future for Netflix and its recommendation algorithm.